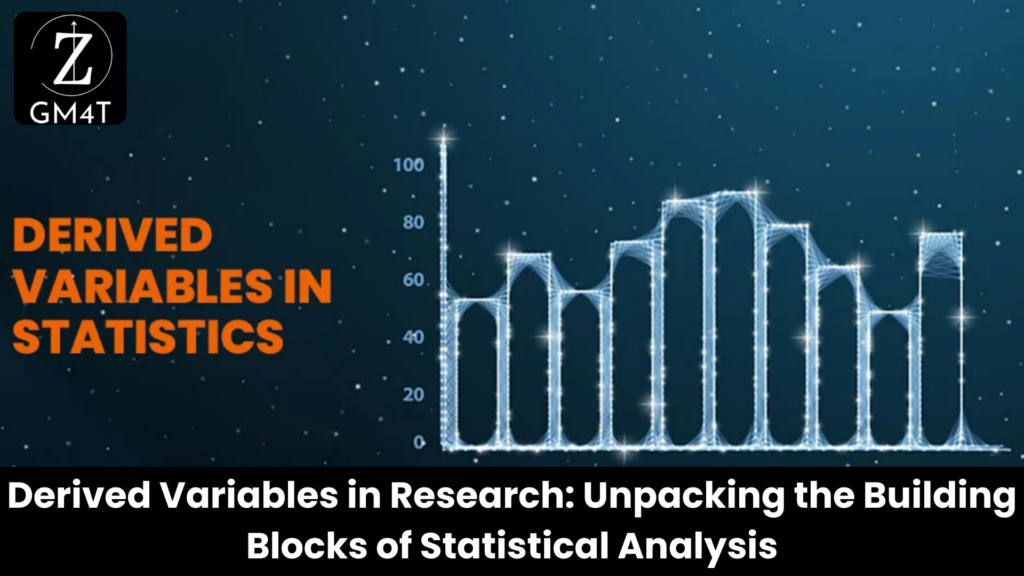
Derived variables are an essential aspect of data analysis in research. While raw data forms the foundation, derived variables enhance clarity and facilitate meaningful analysis by transforming or categorizing data. In this blog, we’ll explore the various types of derived variables, their applications, and practical examples to help researchers understand their value.
What Are Derived Variables?
Derived variables are those created from the original recorded data for analytical purposes. They allow researchers to uncover patterns, compare populations, or meet the assumptions of statistical models. Here’s a breakdown of the key types:
1. Calculated or Categorized Variables
Derived variables often result from simple calculations or categorizations of recorded data.
Examples:
• Age at Diagnosis:
Researchers commonly calculate a patient’s age at diagnosis by finding the number of days between their date of birth and date of diagnosis and dividing this by 365.25 (accounting for leap years).
Further categorization might group patients into age groups (e.g., 30–39, 40–49), which is an ordered categorical variable.
• Income Groups:
By dividing a sample’s observed income range into quintiles, researchers create a new variable, ‘income group,’ where ‘1’ represents the least affluent and ‘5’ the most affluent.
• BMI Categories:
BMI is calculated as weight (kg) divided by height (m²). It is often categorized into groups like:
• <16 kg/m²: Malnourished
• 16–18.5 kg/m²: Underweight
• 18.5–24.9 kg/m²: Normal weight
• 25–29.9 kg/m²: Overweight
• ≥30 kg/m²: Obese
Unlike income groups, BMI categories use universally accepted thresholds.
Key Insight:
Categorized variables make analysis easier by organizing data into manageable groups. However, the method of categorization (data-specific vs. standardized thresholds) affects interpretation.
2. Variables Based on Threshold Values
Derived variables often use predefined thresholds to simplify analysis.
Examples:
• Low Birthweight (LBW):
This binary variable categorizes birthweight as:
• “Yes” (below 2500 g)
• “No” (2500 g or above)
• Vitamin A Status:
Derived from serum retinol levels, this is an ordered categorical variable that classifies individuals into groups like “deficient,” “adequate,” and “excess.”
Key Insight:
Threshold-based variables are particularly useful in medical research, where they can directly inform clinical decision-making.
3. Variables Derived from Reference Curves
When comparing an individual’s data against population norms, derived variables help interpret deviations.
Example:
• Child Growth Monitoring:
A child’s weight and height are plotted against standard growth curves, allowing researchers to assess:
• How the child compares to the average child of the same age.
• Whether growth faltering occurs if the child’s growth curve drops below expected norms.
Key Insight:
Reference curve-based variables provide nuanced insights, helping researchers and clinicians identify anomalies and trends.
4. Transformed Variables
Sometimes, numerical variables need to be transformed to meet the assumptions of statistical models.
Examples of Transformation:
• Logarithmic Transformation:
Replace the value of a variable with its logarithm to stabilize variance or make data conform to normality.
This is commonly used for:
• Incubation periods
• Parasite counts
• Dose levels
• Concentrations of substances
Why Transform?
Statistical methods like regression often assume data follows a specific distribution. Transformations ensure compliance with these assumptions, improving model performance.
Why Derived Variables Matter
Derived variables are more than just mathematical conveniences—they shape the analysis process and enable deeper insights. Here’s why they’re indispensable:
• Simplifying Analysis: Derived variables like age groups and income quintiles make complex data more accessible.
• Improving Comparability: Reference curve-based variables enable direct comparisons against population norms.
• Enhancing Statistical Robustness: Transformed variables help meet statistical assumptions, ensuring accurate results.
Practical Applications in Research
1. Epidemiology:
Categorizing BMI into standard thresholds allows for global comparisons of obesity prevalence.
2. Public Health:
Using income quintiles highlights disparities in healthcare access.
3. Clinical Trials:
Low birthweight categories guide interventions for neonatal health.
4. Biomedical Research:
Logarithmic transformations stabilize skewed data for accurate modeling of parasite counts or substance concentrations.
Final Thoughts: Making the Most of Derived Variables
Derived variables are vital tools for researchers. From simplifying raw data to ensuring statistical rigor, they enhance every stage of the analysis process. By understanding the different types and their applications, you can wield these tools effectively in your research.
Engage With Us: How do you use derived variables in your research? Share your experiences and examples in the comments below. Let’s explore how these variables can transform raw data into actionable insights!